A break-through of Transformers
- Nyan Swan Aung
- Jan 20, 2021
- 1 min read
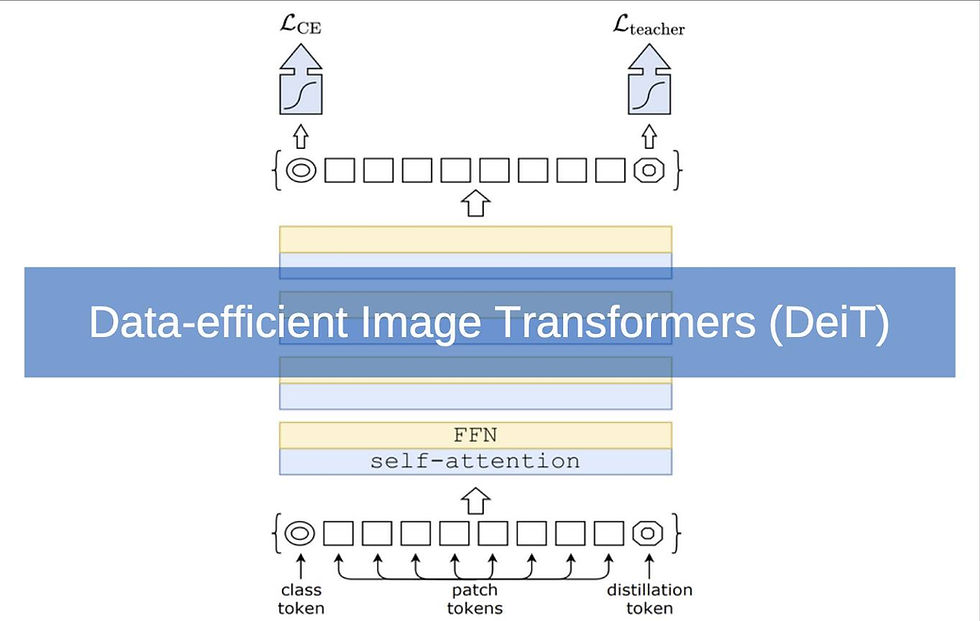
Everyone has been using the dominant Convolutional Neural Networks(CNN) based state-of-the-art (SOTA) models for various computer vision applications mainly for image classification, object detection and segmentation.
Ever wonder using Transformers (which produce SOTA results in NLP, Machine Translation, Speech Recognition, etc) as an enhancement to computer vision as out of the box?
Facebook AI Team just developed a new Transformer based object detection architecture to leverage computer vision called DeiT (Data-efficient image Transformers) in 2020. DeiT requires far less data and computing resources to produce a high performance image classification, that is, rather than needing hundreds of millions of images, DeiT can be trained effectively with 1.2 million images.
So what makes this Transformer based DeiT unique for classifying images?
Firstly, its training strategy includes data augmentation, optimisation and regularization in order to simulate training on much larger dataset.
Secondly FB AI Team modified the Transformer architecture to enable native distillation. They added a distillation token to the Transformer which interacts with the classification vector and image component tokens through the attention layers. The objective of this distillation token is to learn from the teacher model (a CNN).
[Note: Current implementation is only available in Pytorch]
Benchmark Results on open source datasets (ImageNet, CIFAR, Flowers-102, Oxford 102 Flowers, Stanford Cars) https://paperswithcode.com/.../training-data-efficient...
Comments